Characterizing Post-Fire Burn Severity And Vegetation Recovery At High Spatial And Temporal Resolutions Using Basal Area Measurements
USGS scientists are studying the effects of wildfires on forests and developing models to predict forest recovery potential after fires. They have collected and analyzed data from several recent California wildfires, including the Dixie, Caldor, KNP Complex, Carr, and Mosquito fires. Researchers are using the data to analyze changes in basal area (the cross-sectional area of tree trunks) to measure fire severity and recovery potential and to identify the presence or absence of live trees after fires to quantify forest recovery potential.
The goal of the vegetation dynamics research team of The Post-Fire Hazards Impacts to Resources and Ecosystems (PHIRE): Support for Response, Recovery, and Mitigation project is to characterize burn severity and vegetation recovery at high spatial and temporal resolutions in the Caldor, Dixie, and KNP complex Fires. This information will inform water and landslide hazard objectives and to develop a set of tools that aid strategies by land managers in efforts to recover desirable vegetation. Critical science questions that will be addressed by the vegetation dynamics team include:
- Characterizing fine-scale variability in fire severity,
- describing patterns in delayed tree mortality, and
- integrating these findings with other project branches.
Identifying available datasets
We developed partnerships to acquire pre- and post-fire basal area measurements from >300 plots to model surviving basal area as a function of burn severity indices (Relative difference Normalized Burn Ratio (RdNBR), difference Normalized Burn Ratio (dNBR), and Relative Burn Ratio (RBR)). We have also developed new data to support our work. We acquired precise location information for permanent plot data in the Dixie Fire. Technicians created training data from NAIP imagery, manually scoring the presence or absence of live trees in more than 6,000 points across the Dixie, Caldor and KNP Fires.
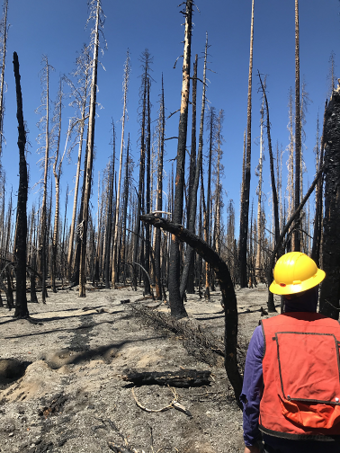
Field data collection
We developed a field protocol to collect validation data for our high-resolution model of post-fire surviving trees and collected > 250 validation points stratified across diverse post-fire conditions in areas burned one year ago (Mosquito Fire) and two years ago (Caldor Fire). We have also collected tree locations from permanent forest plots that burned in the KNP Fire at Sequoia National Park and plot locations from permanent plots that burned in the Dixie Fire using a high-resolution GPS.
Data analysis
We have taken a two-pronged approach to characterize fire severity. One analysis considers basal area change following fire to measure fire severity, which will be used to characterize both fire effects and recovery potential. The second analysis quantifies forest recovery potential by identifying the presence of live trees following fire. Both analyses consider data from the Dixie, Caldor, Carr, and KNP Complex Fires.
Basal area changes following fire
We used pre- and post-fire basal area measurements from small (15-30cm), medium (30-50cm) and large (>50cm) trees in 585 plots to model the proportion surviving basal area by size class as a function of three different burn indices: dNBR, RdNBR, and RBR. We calculated the burn indices using pre and post-fire temporal composites of 10m resolution Sentinel-2 data. We processed the temporal composites using Google Earth Engine, and included all images from the month of June for the year before (pre-fire) and after (post-fire) the fire. We masked all scenes with 10% or more cloud percentage. We parameterized Bayesian zero-one inflated beta regression models (ZOIB) for the proportion basal area change as a function of the area-weighted mean of each burn severity index for each plot and size class, subsetting by subplot where available. We included an interaction term between size class and burn index to determine if the relationship between burn indices and basal area mortality varied by size class. We compared the models using the different indices using Leave One Out cross validation (LOO). The selected model showed increasing basal area mortality with increasing RBR. Additionally, the model also showed that mortality of large trees increased more slowly with increasing RBR than the smaller size classes, suggesting that models incorporating size class may more accurately represent mortality in large trees than when size class is ignored. We then used the fitted model to predict the proportion surviving basal area in each fire using the RBR rasters we created.
Live tree presence/absence
We developed a workflow in R to use Google Earth Engine to download Sentinel-2 10 m resolution monthly composite means of post fire images for the Dixie, Caldor and KNP Fires. Using these images, we developed Random Forest models using land surface reflectance of all Sentinel-2 bands to predict live tree locations performing with ~80% accuracy. These models were then used to produce preliminary live tree maps across the fires. We are now testing the applicability of the models to new (e.g., future) fires, using validation data from both manual imagery interpretation and field data. We are developing a manuscript that describes this work.
Next steps
In FY2024, the team will complete the analyses described above and develop accompanying data releases. We will use the fitted models to assess the drivers of delayed mortality and post-fire regeneration potential. We will develop peer-reviewed manuscripts for these analyses with submission expected in FY2024.
Funding
Funding for this project is provided by the Robert T. Stafford Disaster Relief and Emergency Assistance Act (42 U.S.C. 5121 et seq.) and supplemental funding acts for Federal disaster relief activities. Through this funding USGS supports recovery efforts in declared natural disaster areas, to aid recovery efforts from widespread wildfires, devastating hurricanes, prolonged volcanic eruptions, and damaging earthquakes. This enables USGS to repair and replace equipment and facilities, collect high-resolution elevation data, and conduct scientific studies and assessments to support recovery and rebuilding decisions. The methods developed in this study purposely used freely available remotely sensed imagery and could be applied to future fires without substantial costs.
Post-Fire Hazards Impacts to Resources and Ecosystems (PHIRE): Support for Response, Recovery, and Mitigation
USGS scientists are studying the effects of wildfires on forests and developing models to predict forest recovery potential after fires. They have collected and analyzed data from several recent California wildfires, including the Dixie, Caldor, KNP Complex, Carr, and Mosquito fires. Researchers are using the data to analyze changes in basal area (the cross-sectional area of tree trunks) to measure fire severity and recovery potential and to identify the presence or absence of live trees after fires to quantify forest recovery potential.
The goal of the vegetation dynamics research team of The Post-Fire Hazards Impacts to Resources and Ecosystems (PHIRE): Support for Response, Recovery, and Mitigation project is to characterize burn severity and vegetation recovery at high spatial and temporal resolutions in the Caldor, Dixie, and KNP complex Fires. This information will inform water and landslide hazard objectives and to develop a set of tools that aid strategies by land managers in efforts to recover desirable vegetation. Critical science questions that will be addressed by the vegetation dynamics team include:
- Characterizing fine-scale variability in fire severity,
- describing patterns in delayed tree mortality, and
- integrating these findings with other project branches.
Identifying available datasets
We developed partnerships to acquire pre- and post-fire basal area measurements from >300 plots to model surviving basal area as a function of burn severity indices (Relative difference Normalized Burn Ratio (RdNBR), difference Normalized Burn Ratio (dNBR), and Relative Burn Ratio (RBR)). We have also developed new data to support our work. We acquired precise location information for permanent plot data in the Dixie Fire. Technicians created training data from NAIP imagery, manually scoring the presence or absence of live trees in more than 6,000 points across the Dixie, Caldor and KNP Fires.
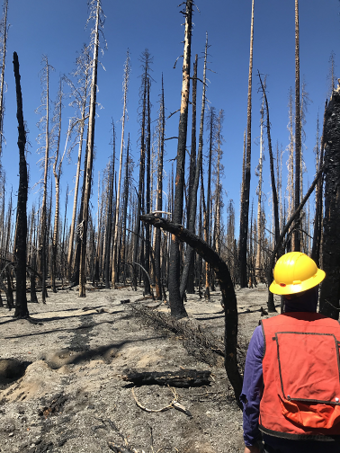
Field data collection
We developed a field protocol to collect validation data for our high-resolution model of post-fire surviving trees and collected > 250 validation points stratified across diverse post-fire conditions in areas burned one year ago (Mosquito Fire) and two years ago (Caldor Fire). We have also collected tree locations from permanent forest plots that burned in the KNP Fire at Sequoia National Park and plot locations from permanent plots that burned in the Dixie Fire using a high-resolution GPS.
Data analysis
We have taken a two-pronged approach to characterize fire severity. One analysis considers basal area change following fire to measure fire severity, which will be used to characterize both fire effects and recovery potential. The second analysis quantifies forest recovery potential by identifying the presence of live trees following fire. Both analyses consider data from the Dixie, Caldor, Carr, and KNP Complex Fires.
Basal area changes following fire
We used pre- and post-fire basal area measurements from small (15-30cm), medium (30-50cm) and large (>50cm) trees in 585 plots to model the proportion surviving basal area by size class as a function of three different burn indices: dNBR, RdNBR, and RBR. We calculated the burn indices using pre and post-fire temporal composites of 10m resolution Sentinel-2 data. We processed the temporal composites using Google Earth Engine, and included all images from the month of June for the year before (pre-fire) and after (post-fire) the fire. We masked all scenes with 10% or more cloud percentage. We parameterized Bayesian zero-one inflated beta regression models (ZOIB) for the proportion basal area change as a function of the area-weighted mean of each burn severity index for each plot and size class, subsetting by subplot where available. We included an interaction term between size class and burn index to determine if the relationship between burn indices and basal area mortality varied by size class. We compared the models using the different indices using Leave One Out cross validation (LOO). The selected model showed increasing basal area mortality with increasing RBR. Additionally, the model also showed that mortality of large trees increased more slowly with increasing RBR than the smaller size classes, suggesting that models incorporating size class may more accurately represent mortality in large trees than when size class is ignored. We then used the fitted model to predict the proportion surviving basal area in each fire using the RBR rasters we created.
Live tree presence/absence
We developed a workflow in R to use Google Earth Engine to download Sentinel-2 10 m resolution monthly composite means of post fire images for the Dixie, Caldor and KNP Fires. Using these images, we developed Random Forest models using land surface reflectance of all Sentinel-2 bands to predict live tree locations performing with ~80% accuracy. These models were then used to produce preliminary live tree maps across the fires. We are now testing the applicability of the models to new (e.g., future) fires, using validation data from both manual imagery interpretation and field data. We are developing a manuscript that describes this work.
Next steps
In FY2024, the team will complete the analyses described above and develop accompanying data releases. We will use the fitted models to assess the drivers of delayed mortality and post-fire regeneration potential. We will develop peer-reviewed manuscripts for these analyses with submission expected in FY2024.
Funding
Funding for this project is provided by the Robert T. Stafford Disaster Relief and Emergency Assistance Act (42 U.S.C. 5121 et seq.) and supplemental funding acts for Federal disaster relief activities. Through this funding USGS supports recovery efforts in declared natural disaster areas, to aid recovery efforts from widespread wildfires, devastating hurricanes, prolonged volcanic eruptions, and damaging earthquakes. This enables USGS to repair and replace equipment and facilities, collect high-resolution elevation data, and conduct scientific studies and assessments to support recovery and rebuilding decisions. The methods developed in this study purposely used freely available remotely sensed imagery and could be applied to future fires without substantial costs.