Environmental streamflows in the United States: historical patterns and predictions
The term environmental streamflows refers to the magnitude, frequency, seasonal timing, duration, and rate of change of streamflows needed to sustain freshwater and estuary ecosystems and human wellbeing. It is important that environmental streamflow assessments by water managers consider changes in climate, land use, and water management; this cannot be done effectively without understanding variability and changes in environmental streamflows over time and space. A limited amount of research of this type has been published. Estimates of environmental streamflows also are needed for ungaged streams; machine-learning methods are likely useful for this. We will analyze historical change and variability at hundreds of streamflow gages across the United States for a suite of environmental streamflows and use machine-learning methods to estimate environmental streamflows for thousands of ungaged stream reaches. The current project builds on previous projects that focused on a limited number of environmental flows, such as the magnitude of low flows and flood flows.
Statement of Problem:
It is important that environmental streamflow assessments consider climatic and watershed changes; however, this requires understanding historical variability and changes in environmental streamflows. There is no known published work that quantifies the variability and change for a full suite of environmental streamflows in the United States at the regional or continental scale. There are many commonly used environmental streamflows that have not been systematically analyzed, such as the number and duration of high and low pulses in each year and measures of the rate of change of streamflows from day to day.
Thousands of streams within the United States do not have directly measured streamflow values (normally from USGS streamgages). Models to predict environmental streamflows for ungaged streams are an important tool for water resource managers. Data-driven models using machine-learning methods are increasingly being applied in hydrologic science and achieve predictive skill comparable to, or greater than, traditional process-based modeling methods. It would be very useful to know whether machine-learning methods can better predict environmental streamflows for ungaged rivers than traditional methods.
Why this Research is Important:
Managers use estimates of environmental streamflows as targets to approximate various aspects of natural streamflows. They can reach these targets by controlling flow from reservoirs. Approximating natural flow promotes the health of fish and other aquatic species. Little is known, however, about the historical variability of environmental flows over time and space. Our work will substantially help define this variability and provide important context for managing streamflow now and in the future.
Objective(s):
- Characterize temporal and spatial patterns for a suite of environmental streamflows across the conterminous United States based on streamflow data from hundreds of long-term streamflow gages. The environmental streamflows will include metrics of streamflow magnitude, frequency, seasonal timing, duration, and rate of change.
- Develop machine-learning models to estimate environmental streamflows for ungaged streams across the conterminous United States and compare results to streamflows estimated from traditional methods.
- In addition, we will focus on environmental flows specifically relevant to migratory marine fish in selected regions of the U.S.
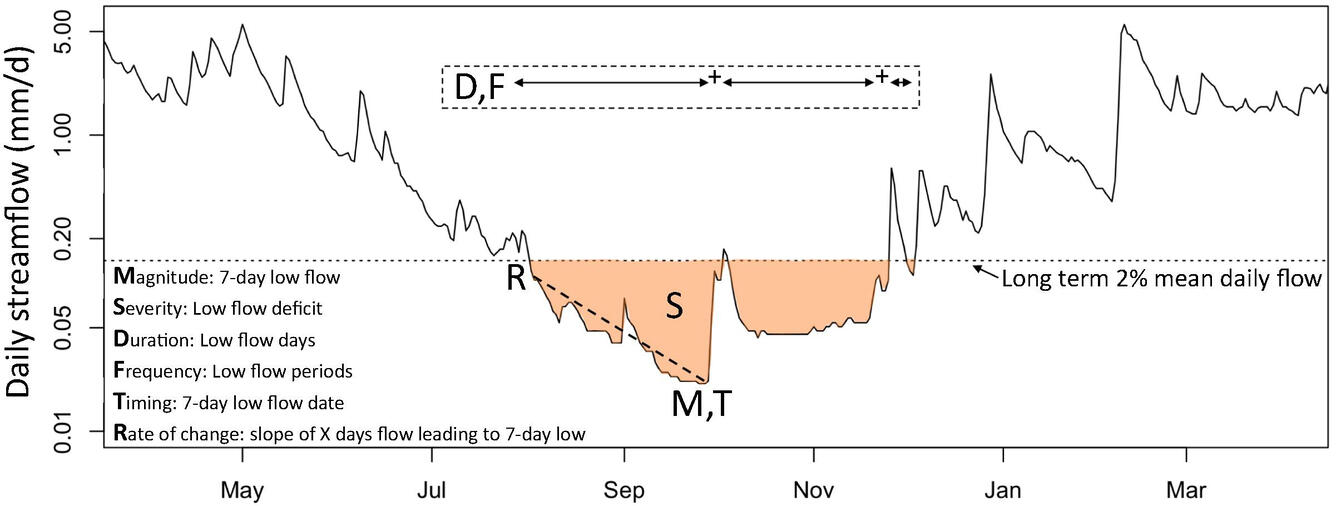
Methods:
We will use trend-testing methods to analyze changes over time and space in environmental streamflows and analyze how much variability is explained by atmosphere-ocean patterns such as the Pacific Decadal Oscillation and the North Atlantic Oscillation. We will consider how changes in climate, reservoir regulation, and urban and agricultural land uses have impacted environmental streamflows.
We will develop machine learning models using variables such as basin soil characteristics and land use, precipitation, and temperature data. Multiple methods will be considered and evaluated.
The Influence of Climatic, Watershed, and Water-use Changes on Extreme Low Streamflows in the United States
Climate-driven variability in the occurrence of major floods across North America and Europe
Trends in snowmelt-related streamflow timing in the conterminous United States
Reference hydrologic networks I. The status and potential future directions of national reference hydrologic networks for detecting trends
Historical summer base flow and stormflow trends for New England rivers
Streamflow changes in Alaska between the cool phase (1947–1976) and the warm phase (1977–2006) of the Pacific Decadal Oscillation: The influence of glaciers
The term environmental streamflows refers to the magnitude, frequency, seasonal timing, duration, and rate of change of streamflows needed to sustain freshwater and estuary ecosystems and human wellbeing. It is important that environmental streamflow assessments by water managers consider changes in climate, land use, and water management; this cannot be done effectively without understanding variability and changes in environmental streamflows over time and space. A limited amount of research of this type has been published. Estimates of environmental streamflows also are needed for ungaged streams; machine-learning methods are likely useful for this. We will analyze historical change and variability at hundreds of streamflow gages across the United States for a suite of environmental streamflows and use machine-learning methods to estimate environmental streamflows for thousands of ungaged stream reaches. The current project builds on previous projects that focused on a limited number of environmental flows, such as the magnitude of low flows and flood flows.
Statement of Problem:
It is important that environmental streamflow assessments consider climatic and watershed changes; however, this requires understanding historical variability and changes in environmental streamflows. There is no known published work that quantifies the variability and change for a full suite of environmental streamflows in the United States at the regional or continental scale. There are many commonly used environmental streamflows that have not been systematically analyzed, such as the number and duration of high and low pulses in each year and measures of the rate of change of streamflows from day to day.
Thousands of streams within the United States do not have directly measured streamflow values (normally from USGS streamgages). Models to predict environmental streamflows for ungaged streams are an important tool for water resource managers. Data-driven models using machine-learning methods are increasingly being applied in hydrologic science and achieve predictive skill comparable to, or greater than, traditional process-based modeling methods. It would be very useful to know whether machine-learning methods can better predict environmental streamflows for ungaged rivers than traditional methods.
Why this Research is Important:
Managers use estimates of environmental streamflows as targets to approximate various aspects of natural streamflows. They can reach these targets by controlling flow from reservoirs. Approximating natural flow promotes the health of fish and other aquatic species. Little is known, however, about the historical variability of environmental flows over time and space. Our work will substantially help define this variability and provide important context for managing streamflow now and in the future.
Objective(s):
- Characterize temporal and spatial patterns for a suite of environmental streamflows across the conterminous United States based on streamflow data from hundreds of long-term streamflow gages. The environmental streamflows will include metrics of streamflow magnitude, frequency, seasonal timing, duration, and rate of change.
- Develop machine-learning models to estimate environmental streamflows for ungaged streams across the conterminous United States and compare results to streamflows estimated from traditional methods.
- In addition, we will focus on environmental flows specifically relevant to migratory marine fish in selected regions of the U.S.
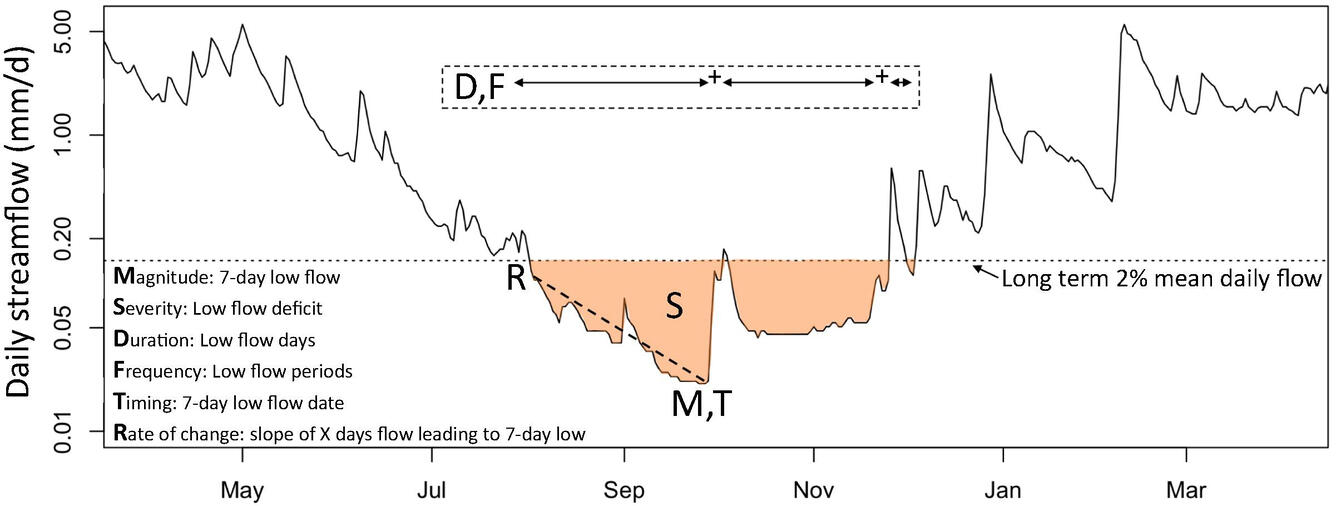
Methods:
We will use trend-testing methods to analyze changes over time and space in environmental streamflows and analyze how much variability is explained by atmosphere-ocean patterns such as the Pacific Decadal Oscillation and the North Atlantic Oscillation. We will consider how changes in climate, reservoir regulation, and urban and agricultural land uses have impacted environmental streamflows.
We will develop machine learning models using variables such as basin soil characteristics and land use, precipitation, and temperature data. Multiple methods will be considered and evaluated.